The Generative AI Industrial Revolution
Generative AI may prove even more divisive to society than the Industrial Revolution. There are many questions well worth examining regarding gen AI’s impact on the streaming industry, the media and entertainment world, and more. In this article, I’m going to focus on a few streaming use cases in the hope of providing a broader perspective on the changes happening in our world now and those we can expect going forward.
At Streaming Media Connect in August 2023, I moderated a session on How Generative AI Will Impact Media Technologies. A year later, the conversations are becoming much more grounded and nuanced. Media customers are willing to do prototypes for the projects for which they want to use gen AI to test out ideas. This is a large step forward, says a rep from one midsize enterprise.
But let’s start out by defining what gen AI is—and what it’s not—and discussing how users typically interact with gen AI models.
AI vs. Generative AI
There’s generally some confusion about the differences between AI/machine learning and gen AI. We have to be clear about separating machine learning from gen AI since they are very different. The distinction is that gen AI is trained on extensive amounts of data to duplicate human output. Whether it’s code, graphics, text, video, or audio, the interaction with the system uses natural language processing and can create output based on training knowledge. Machine learning is used for understanding and predicting based on an existing body of data.
Welcome to Your Chatbot
The interaction layer for many, if not all, gen AI projects is accomplished by chatbot, in which input is either speech or text. This prompt engineering (structuring instructions to required output) is now replacing the traditional user interface on existing products. Configuring a workflow in this way can save time and—in theory—requires less expertise in using a specific application. However, producing the desired output still requires skills, just different skills. In other instances, the use cases create an entirely new way of doing something. Meanwhile, the gen AI model learns from the data you’re providing and has the potential to suggest the best way to get from A to B, handling the many nuances along the way.
“The foundational apps I see coming out now are really guided assistance, augmenting the use of a piece of software,” says a rep from one of the larger vendors. “The next step would be automating automation, whether it’s specific tasks that you can do conversationally or whether it’s a complete workflow.”
Accuracy
Before launching into use cases, I wanted to find out what types of questions vendors were receiving from media companies.
Globant has engineering staff and media technology products in use with many of the well-known large media companies. According to Luciano Marcos Escudero, the company’s VP of media engineering, customers want to know how gen AI works with their data and how accurate the output will be. An example might be that they could use metadata associated with a content library for creating a recommendation service. An inaccurate result might be an improper classification of a movie genre, not associating an actor with all of their films, or some other hallucination when the system makes something up. “We try to build some sort of correlation with the input and the output, but it’s not 100%,” says Escudero. “We throw things to a model that has been training and self-training again. The reasoning based on the data is not always accurate.” Nor is it clear how that response was generated. Both of these things can make customers very uncomfortable. In all of the use cases I discuss here, vendors put a rule engine (or prompt engineering to restrict output) on top to filter out inaccurate responses.
Large language models (LLMs) out in the wild today are trained on general data that’s not specific to media and entertainment. Naveen Narayanan, Quickplay’s head of product innovation and strategy, says his company “bound [its LLM] to questions related to media and entertainment catalogs, fine-tuning the gen AI large language model.” The result, he notes, was that the model was able “to answer on a specific question and not answer certain other questions that were not on media. Fine-tuning took us close to 3 months of different prompts and different boundaries. Some of the problems that we [encountered] when dealing with these LLMs were [how they] handle things like consistency, accuracy. … LLMs are not consistent. Sometimes they give you [responses] in certain formats, sometimes they don’t. There is some randomness to it.”
After 3 months, this fine-tuning process yielded a first version that was fairly usable, with a chat-based interface that understood the history of what the user was trying to find. This AI-based Quickplay Media Companion helped users to search and discover contents through a voice-based natural language interface.

Quickplay Media Companion
But It’s My Data
A question that comes up often, Narayanan says, is “‘How do I ensure that what you’re doing is not feeding back to the model and getting the benefit of all of my data?’ The proprietary models that the AWSs and the Googles of the world have launched have a master model that is available. They instantiate that model into a local instance, and all of the training for that particular model is residing within that local instance. It does not go back to the core base model.” The base model, Narayanan maintains, is not learning from the proprietary data that any of the customers have.
“You can easily upgrade the model in your local project, still maintain all of your fine-tuning, and get the benefit” of that without having to worry that you’re “giving any of the proprietary information back to the core base model,” Narayanan states. “That is a big architectural decision that these large cloud vendors have made to tackle this enterprise concern.” The theory is that the base model is getting smarter from data from the open internet, but that enterprise data, for better or worse, is not contributing to this knowledge. Quickplay’s instance of the model, trained within its enterprise, is operating within a closed loop of information.
Do clients always insist on closed-loop gen AI models? Sports organizations seem more ready to jump into using gen AI and are more flexible about how their data is used. “[Sports] are a little bit more flexible on using external models that are already trained,” says Escudero. “We are actually training models with their data too.”
By contrast, Escudero says, “Media [companies] are completely the opposite. Media groups are not that open to external models. They want to run models with their specific IP. Putting their IP into a model has security and governance processes, and we need [to provide] a lot of documentation about how that is going to be processed.”
Cost
There are two ways to look at gen AI costs: what you save and what you spend. “Right now, a lot of our big customers are looking to leverage gen AI at scale to do things that they’ve traditionally been doing, but with a lot more cost optimization and better go-to-market speed,” says Amit Tank, senior director of solutions architecture at MediaKind. “For medium-to-smaller-size customers and prospective customers, what we’re seeing is that they want us to help them leverage [gen AI] to differentiate by offering certain features that are otherwise not feasible.” This includes more easily managing configurations for all encoding devices or providing custom clips, depending on user preference.
While one conversation is about what you can save, another concerns model management and oversight, which is especially relevant if you’re planning on replacing a person with a gen AI function. This has been an underlying theme in many of these conversations.
“One of the initial questions we get is, ‘How much is it going to cost me’” to run an AI project, says Escudero. Globant clients typically want to know, “How much is it going to cost me running one asset for 2 hours, on a daily basis, multiple times?” Other questions he hears are, “What’s the quick time to value? What are the things that can be implemented within a month or 2 that can really generate value?”
Magic Content Creation
Our first use case involves virtual production of highlight videos for live or VOD sports content. Using AI-enhanced or -generated metadata, Linius searches through video, sorts typically 10- or 20-second clips, and builds a video from this for either fans or internal content curation teams. The company specializes in AI-enhanced personalized video experiences, particularly for sports. “We’re using a combination of machine vision to identify players, actions, noise analysis, and commentator sentiment to rank and prioritize moments in the game, and OCR to interpret on-screen data,” says Linius CEO James Brennan.
You could literally ask the model to produce a video clip of all of the left-handed pitches in a baseball game or the goals a particular hockey player scored in Toronto only in the month of March. “Our system accesses the original videos,” notes Brennan. “We don’t ever copy, move, process, or restore those videos. We’re just creating a lightweight data representation and then using the AI to manipulate that data.”
Explaining how the process works, Brennan continues, “Gen AI interprets all data to determine what should be in a highlight reel, writes a summary, creates a commentator script (for text or converted to speech), and then generates the API search queries, which creates the video on-the-fly.” Linius uses standard HLS, MP4 content, and AI to standardize size (16:9, 9:16, 1:1), resolution, etc. “To publish, I copy this URL, paste that into my web CMS, and the video is live.”
But the video viewers see is not a discrete video file. “I’m publishing an instruction set to tell the system, ‘Go pull these different [clips] on-the-fly,’” notes Brennan. Linius’ Whizzard Captivate solution is available by UI or API.

Linius Captivate in action
Stringr provides WeatherGen, a platform that deploys gen AI models to create customized news and weather programming. “About a year ago, we began developing a suite of solutions that leveraged gen AI to create automated video content. Our thinking was that there’s too much risk to call it hard news if there are hallucinations, but if you’re focused on things that have very, very clean data, then there’s an opportunity,” says Stringr COO and chief product officer Brian McNeill.
Stringr began with weather forecasts using content from the National Weather Service. “We parsed that through our own systems, and then we sent that into a variety of large language models to generate a script. That script comes back and goes into a different gen AI model to make a voiceover. We stitch that together into a video with motion graphics to make a weather forecast. Because this is entirely automated, it allows our customers [local media companies] to create voiced video weather forecasts for markets where it might not have made economic sense to actually have a reporter.”
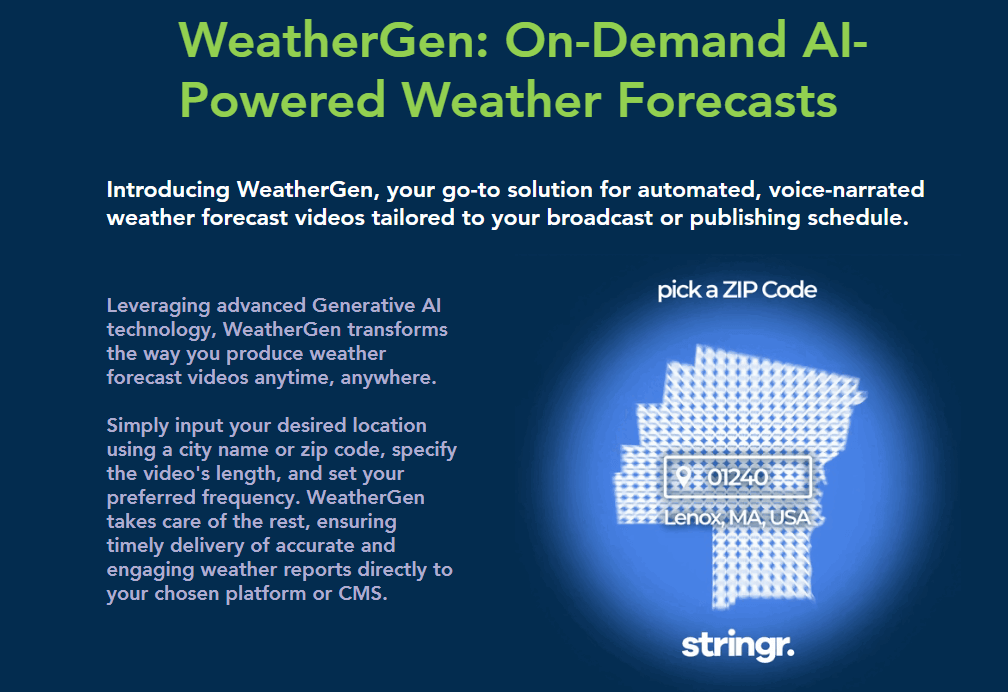
Stringr WeatherGen
The typical length for these forecasts is 30, 60, or 90 seconds at 720p. Stringr is producing content in English and Spanish. Cox Media Group has a FAST channel that uses this, and in August, three other larger station groups signed contracts to use Stringr for their digital properties. Much as with Linius’ product, customers either use a UI to configure their streams, or they can do bulk programming via API.
Here’s another live use case. “The big problem with live is the processing time. We are not talking about seconds; we’re talking about minutes or even hours. Because of that, it is not worth it to do live at this stage,” says Globant’s Escudero. “If we [process the video] to create metadata of all content, audio, etc., it’s going to take time and money. If we go live, it’s even worse, because the latency is much worse than any 4K transcoding today.”
One issue with attempting to deploy gen AI to customize live streams, Escudero explains, is that it means “trying to contextualize key moments not based on the video processing or image processing, but more on metadata.” Sports metadata, created in the stadium in real time by a real person, will track key moments: a fight, a yellow card, a goal, or a time-out. “We’re trying to develop a key and identification based on that metadata without analyzing the video,” he says. This can trigger the creation of a “high-interest” automated clip without requiring human video crew members to produce it.
Your Network Wants to Talk to You About Something Serious
One gen AI application that offers a boon to streaming users, according to Jon Alexander, Akamai’s VP of products, is the ability to have more natural, conversational interactions with the data in their systems. “We have a reporting application today where we’ve introduced a chat interface where you can ask questions of your data,” he says. “We’re primarily using gen AI as a natural language interface for a customer to say, ‘Hey, tell me what’s going on in this segment of my network,’ or ‘What type of threats am I seeing over here?’ One of the areas personally that I feel is most interesting about gen AI is actually a user interface improvement. You can have more conversational interaction with your data with a system versus pushing buttons or writing very arcane instructions in some kind of predefined language.”
The output, much like Excel, can display the data in a number of formats, from table to line chart to bar chart. If you don’t like one output, you can request another.
This gen AI implementation took an open source model and fine-tuned it based on Akamai’s security solution. It can understand prompts in English, French, German, and Spanish, for starters. “It’s a chat interface that allows you to interact with your security data in the Akamai products,” says Alexander. This was designed for security engineers or site reliability engineers—technical users who are running infrastructure or application developers who are accountable for the service that’s being supported. “They understand our web application firewall, bot management, or our micro segmentation services, and they’re using this gen AI capability to understand it better,” he notes.
How to Glow Up a Pipeline
“For most of the large sports customers or TV operators, one of the biggest pain points is setting up the flows that take their video through that entire live production video pipeline and then ultimately send it out to the end consumers,” says MediaKind’s Tank. “It is costly in terms of labor, it is error-prone, and the risk is that a mistake can take you offline.”
To address this problem, at NAB 2024, MediaKind introduced a new AI-driven pipeline management tool called Fleets & Flows. It has a natural language processing layer to enable video engineers to talk to their encoders. “Previously, it used to take an operator’s team 50 to maybe 60 clicks on five different screens to configure five or 10 different devices,” Tank says. “Now they can simply type, ‘I’m located in New Jersey, and I want to get this channel up and running.’”

MediaKind Fleets & Flows
What’s more, Tank adds, “An operator customer can manage all encoding devices, like the devices which accept the contribution feed, and then in turn send that feed uplink to the platform with the right amount of encoding, etc.”
As great as this sounds, it begs the question, “How do we make sure that the actions taken by the NLP or gen AI capability are accurate and aligned with what the human intent was? Our design and architecture is more human-intent based,” Tank explains. “We train and fine-tune our AI capabilities to understand the intent of that operator.” The AI model populates whatever needs to be configured, and then the operator is able to check to ensure they are happy with the result.
Talking to Your Media Supply Chain
The next gen AI use case involves a company replacing its entire user interface with a chatbot. The premise is that enterprise applications have a bigger learning curve than consumer products, and what better way to flatten that curve than by letting users talk directly to the media supply chain platform?
“Instead of having a requirement for highly trained operators or video engineers to operate our platform, now a very entry-level person can sit down and accomplish the same thing just by typing out what needs to happen,” says Dan Goman, CEO of media supply platform provider Ateliere. This seems to be easier said than done. Who will ensure that the right questions are being asked about what needs to happen?
Typical questions might be: Do I have this content? Do I have all of the right variations? Is it in a state that’s monetizable? Once those issues are resolved, Goman says, the operator can choose to send this to a platform. The system can check about requirements to be modified before doing it by asking, “Would you like to fix this, or can I fix it for you?”
The other use case Ateliere sees is an executive being able to query the costs for a piece of content to be processed. “In studio land, they always ask us what the operational overhead is so they know if they made the right deal or not,” says Goman. “We have all of the costs associated with fulfilling an order, and they could include things like transcoding costs, the cost of packaging that content, and delivery costs to provide a more accurate, more complete cost to business owners,” he says.
Connect AI, the gen AI-augmented, natural language version of Ateliere’s Connect solution is only an option; API access and the interface for the platform will still be available for customers who want a more advanced or traditional way of interacting with the platform.

Uploading assets in Ateliere Connect AI
Gen AI Ad Context
Advertising-centric gen AI use cases take contextual insight based on video, audio, and transcripts analysis (among other things) and find the right placement and the right contextual ad. Previously, FAST programming had many irregular approaches to ad insertion, with some channels running ads every X minutes. “We figured out that there’s a much better way to identify key points where ads can be inserted, and we can also provide the context of the segment before the break point,” says Quickplay’s Narayanan. “We have done a pilot with one of the largest Spanish operators in Latin America.”
The Quickplay AI model performs analysis to determine the right place to put an ad break, as well as requesting a contextually matching ad.
Globant has been working on the same use case. “We built a solution where we receive an asset in a scene and then, after the scene, we break it down in frames and process them to identify what’s in that frame,” says Escudero. Customers use this metadata to look at the context of the content 30 seconds before an ad break so they can pass labels related to what’s happened in the content to the ad server.
I was curious to find out how this data is stored. The metadata lives in Globant’s CMS. Its data model for this can be configured to expand from 10 traditional fields to thousands of different vectors stored along with metadata of that particular asset, Narayanan says. “Some is user-readable, a set of predefined topic keywords in plain text. There is also a process to summarize a fingerprint for every video, and all of the information is stored in vector format. Think of it as a series of numbers that represent what the content is, and it’s easily stored as a set of numbers in our database,” he says.
This metadata can then provide the basis for building out consumer recommendations, transcription, translation, and other use cases that need deeper insight into the video content.
Buy and Sell Side
This next use case is an idea I was always skeptical about for bigger brands, because I figured there were too many brand guidelines to let gen AI create an ad. However, Waymark is targeting local and SMB advertisers and offering its self-serve tech to give smaller advertisers an option for easily designing creative, with multiple versions, in 5 minutes or less. First, it will use any content that exists on the company’s website—the logo, the site copy, and style—and then let users pick a color palette and provide instruction about what kind of storyline to promote.
This product has been white-labeled by Paramount, Scripps, Spectrum Reach, and Fox, and now there are a few international contracts, including with Australia’s Nine and U.K. newspaper publisher National World, PLC. It helped garner more than $200 million in new revenue, giving a much-needed boost to ad revenue in streaming. Spectrum Reach and Waymark generated more than $27 million in revenue alone last year from AI-generated ads for local clients.
“We’ve built a ton of components that create video, like animations, transitions, end cards, and all of those pieces that make a complete video,” says Waymark CEO Alex Persky-Stern. Assets are merged with an AI-generated script, and customers can choose from 150 different voices for narration. The customers can revise as needed or revert back to an older output. Waymark delivers to two different HD formats: one for broadcast and streaming, the other for social.
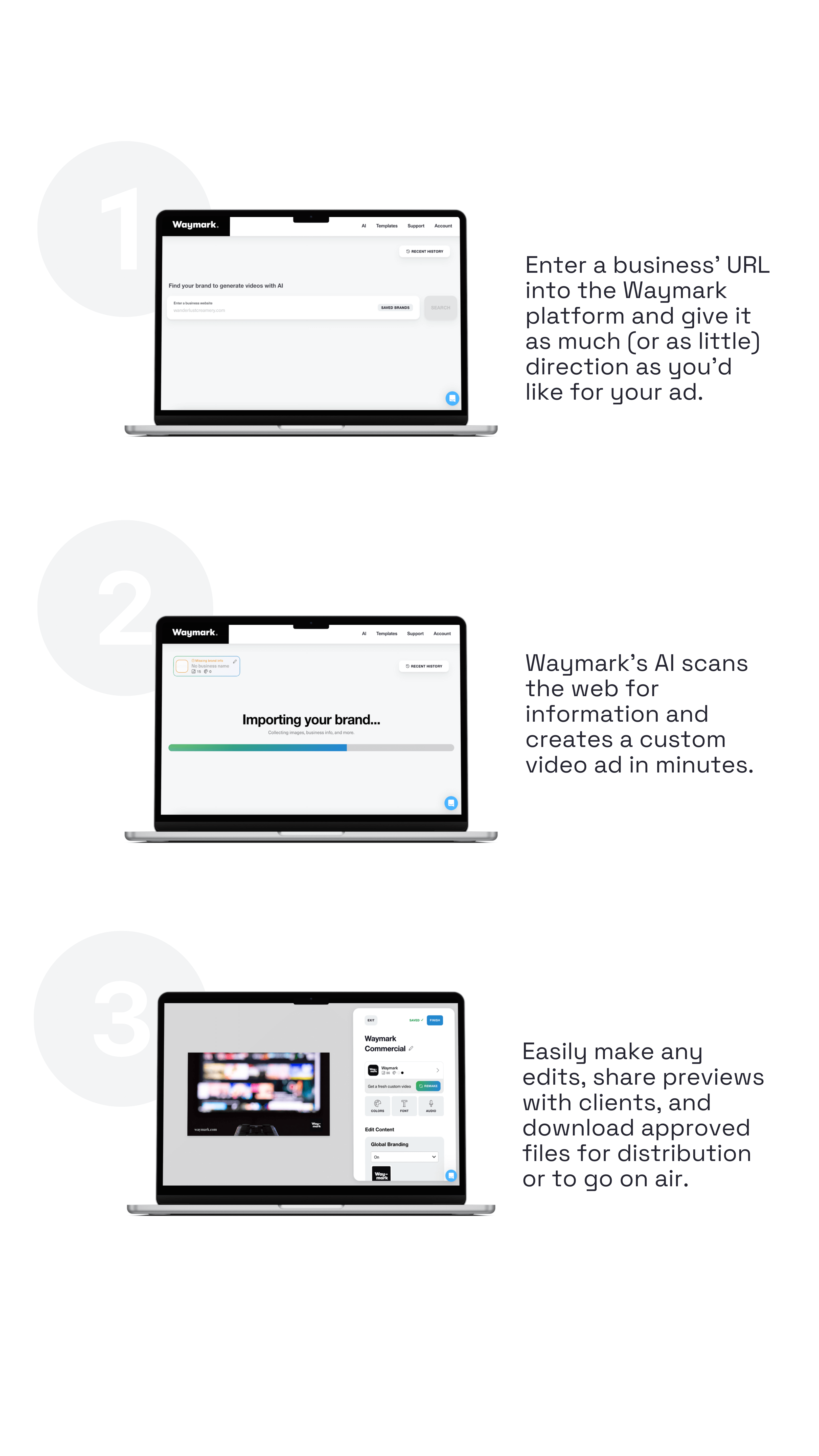
How Waymark works
On the sell side, Operative provides ad management solutions for larger-dollar monetization campaigns. One of the complex puzzles for anybody sitting on a lot of inventories is, “How much of that inventory do I sell direct through my direct sales team? How much do I move to programmatic? How do I optimize inventory for different channels for different advertisers? How do I respond to an unstructured RFP?” says Ben Tatta, chief commercial officer at Operative, whose platform helps many large media companies package and sell advertising.
Media planners can compose an entire plan on where to buy advertising, whom to target, budgeting, and many more details in a conversation with Operative’s chatbot Adeline, and it will populate requirements within the software. You can even upload a voicemail. After a plan is generated, the planner would go through and make modifications.
“A really good plan for BMW starts by pulling all of the historical plans for BMW and/ or [similar] manufacturers,” says Tatta. This increases the hit rate from the pre-AI 50%– 60% average to 80% or above and puts companies with a lot of data in a much better position. “We have a very structured taxonomy for tens of thousands of data elements, which could be how you define an audience, a campaign,” notes Tatta.
When a campaign hits delivery targets, it can be shut down, and the next campaign can begin.
Recommendations
What’s the most common request from customers in the streaming world for what gen AI can deliver? As you might expect, it’s content recommendations.
“We are close to deploying recommendations for our customers in Q4,” says Narayanan. “The way it works is, you have the catalog information that is passed to the gen AI model. You capture the usage information—this person watched this content. The catalog and the consumption information are merged together, based on that mapping, and now you are able to figure out what is the likely content that the user is going to engage next.” They can either optimize on watch time or completion rates for what’s up next.
Being Responsible
What has become clearer in the gen AI era is that talking to your technology will now become much more prevalent. What also is clear is that not all customers understand how AI operates. They believe you put an algorithm in with sample data, and it works. But you have to do A/B testing with AI and expect to iterate through approaches. “You need to test multiple samples that are multiple gen AI algorithms and then find that one with the proper results,” says Escudero. “We need to educate customers about how to think about AI.”
Other important issues (beyond the scope of this article) are copyright within the data that LLMs are trained on and fair representation on a wide range of topics, such as race, sex, age, location, and so many more. Unfortunately, AI models are no less biased than the humans who trained them. Ethics is also another important topic I didn’t look into here, because we needed to first look at use cases. But these other areas are very important, if not more so.
Use cases are what will bring the media and entertainment industry along into what some of the possibilities are, and there are many more than what I covered here (like localization). One company identified five areas to bring value to its customers:
- Increasing monetization opportunities
- Driving user engagement
- Enhancing discovery
- Increasing reach
- Lowering costs
Another company focused on making its product easier to use and controlling costs. A third focused on allowing its customers to make data queries that are specific to their problem.
As in the Industrial Revolution, opinions are very divisive on what gen AI will do to the nature of work. One thing is for sure: You need to both closely parse what your chatbots assume and have wide-ranging conversations about how gen AI will work for your particular use case.
Related Articles
In this expansive interview with Simon Crownshaw, Microsoft's worldwide media and entertainment strategy director, we discuss how Microsoft customers are leveraging generative AI in all stages of the streaming workflow and how they're using it in content delivery and to enhance user experiences in a range of use cases. Crownshaw also digs deep into how Microsoft is building asset management architecture and the critical role metadata plays in effective large-language models (LLMs), maximizing the value of available data.
27 Sep 2024
This article explores the current state of AI in the streaming encoding, delivery, playback, and monetization ecosystems. By understanding the developments and considering key questions when evaluating AI-powered solutions, streaming professionals can make informed decisions about incorporating AI into their video processing pipelines and prepare for the future of AI-driven video technologies.
29 Jul 2024
For Anil Jain, leading the Strategic Consumer Industries team for Google Cloud has meant helping traditional media companies embrace cloud and AI, move to an OpEx-centric business model, and adapt to a more development-oriented mindset in engineering and product management. In this interview, Jain discusses the global shift to cloud-based operations in the media industry, the ways generative AI is disrupting everything from production to packaging to all aspects of the user experience, and what media companies should be afraid of if they're not already.
12 Jun 2024
Following the disruptive rise of ChatGPT, in the coming months and years, if a VC or private equity firm wants to invest their pool of money in a streaming or media technology business, will Generative AI be a must-have component?
26 Jul 2023
Companies and Suppliers Mentioned